Structured and interoperable beneficial ownership data
Benefits of structured and interoperable data
Collecting, storing, and making available BO information as structured data has a number of advantages and benefits in terms of using the information. Broadly, it:
- improves the functionality of BO information;
- reduces the cost of producing, using, and maintaining BO information; and
- has a greater chance of meeting BOT policy goals than unstructured data.
Improved functionality
Box 2. Searching and exploring beneficial ownership data in the Open Ownership Register
The Open Ownership Register (OO Register) allows people to search for beneficial owners and legal entities, and to explore ownership connections across jurisdictions by combining BO data from multiple registers.[e] Ownership structures can be visualised using built-in tools, and data on individuals or entities can be downloaded.
Users are not required to have technical knowledge in order to do this. The OO Register provides this functionality by ingesting data sources from different jurisdictions that publish BO information as structured data.
Because the data is structured, the OO Register is able to provide functionality that some jurisdictions’ own online portals do not. For example, one of the jurisdictions from which the OO Register ingests BO data is the United Kingdom (UK). On the UK’s BO register, it is possible to search for company names, company numbers, and officer names, but not beneficial owners. On the OO Register, it is possible to search the UK data – along with data from other jurisdictions – by beneficial owners’ names.
Structuring data creates information that is predictable: because the structure is predefined, users know what to expect from the data. This makes it easy to work with. Non-technical users can benefit from structured data without ever having to use data directly. Through websites, apps, and other tools, people can access, visualise, and interact with relevant information in a variety of non-technical ways. Structured data can be integrated into both human- and machine-led processes that are either impossible or laborious with unstructured data. These include:
- searching and querying BO data to find records relating to known natural or legal persons, or to find unknown persons that match particular criteria (for example, an address or nationality) (see Box 2);
- bulk analysis of single or combined data sets to find patterns or red flags relating to beneficial ownership, or to assess and improve data quality (see Box 3);
- automation of business processes that involve company ownership information, (for example, supplier onboarding and customer due diligence) (see Box 4);
- converting complex information into user-friendly formats that are appropriate for a given context and audience (for example, a visualisation or summary data table) (see Box 5).
Data standards make it easier to realise the full benefits of this increased functionality because BO data is predictable regardless of its origin, making collaboration and data use in multiple organisations less challenging.
Box 3. Bulk analysis of beneficial ownership data in the United Kingdom
In 2016, the UK became one of the first countries to create a public register of the beneficial owners of companies. The UK register, called the register of People with Significant Control (PSC) was pioneering; it published open, structured data, allowing others to analyse the data in bulk. Bulk analysis was conducted in November 2016 by a consortium of non-governmental organisations.[6] Much was learned from this analysis, both to improve the UK register and for others considering or establishing public BO registers.
The analysis identified a number of issues with the data quality. For example, it showed that allowing people to type their nationality into the relevant field resulted in over 500 spellings of “British”, and 10 beneficial owners listing their nationality as Cornish (a county in England).[7] The analysis also revealed 2,160 beneficial owners provided their date of birth as 2016, and others declared theirs as being as far into the future as 9988. Following the findings of the data analysis exercise in 2016, Companies House (CH) included a prompt within the PSC Register when users provide a date of birth which is below age 16 or over 100, and preventing people entering an age over 110.[8]
Similarly, in 2017, Transparency International and Bellingcat conducted bulk analysis on Scottish Limited Partnerships (SLPs), an obscure entity type that was initially not included within the disclosure requirements. They found that 71% of all SLPs registered in 2016 were controlled by companies based in secrecy jurisdictions.[9] This analysis, combined with reporting on wide-scale abuses involving SLPs,[10] led the UK to bring SLPs within the scope of its disclosure requirements. Subsequent bulk analysis showed a decline in SLP registration, suggesting a deterrent effect of transparency.[11]
Box 4. YouControl: Combining structured beneficial ownership data with other data sources for customer due diligence in Ukraine [12]
YouControl is a Ukrainian company committed to business transparency that has developed an “analytical system for compliance, market analysis, business intelligence, and investigation.”[13] YouControl combines aggregated data from 180 sources – including structured data from Ukraine’s BO register – with its own analysis to provide company profiles with a substantial amount of information, including anything that should raise red flags, such as unpaid taxes, pending lawsuits, and failure to file returns. It uses its own algorithms to calculate financial stability scores and the risk that the company is operating from a fictitious location.
A number of case studies on the website provide examples where companies have saved hundreds of thousands of dollars by using YouControl as part of due diligence processes to identify fraudulent businesses before entering into business with them.[14]
Reduction in cost
Interoperable, structured BO data has significant cost advantages over unstructured information throughout its lifecycle by reducing the cost of:
- publishing, maintaining, and upgrading BO data;
- using BO data;
- realising policy benefits; and
- compliance with disclosure requirements.
Publishing, maintaining, and upgrading beneficial ownership data
The decision to structure BO data generally involves a higher upfront cost that is paid off over time through lower operational costs. Structuring data requires documentation and resolution of underlying ambiguity. Documentation ensures that data is understandable and interpretable by any user by explaining the structure of the data and its contents. For example, documentation can make clear that date fields follow a specific format. Knowing that, for example, the International Organization for Standardization (ISO) 8601 date format is followed (YYYY-MM-DD), means it is clear that 2022-07-11 is 11 July 2022 rather than 7 November 2022.[15]
Unambiguous documentation reduces ongoing maintenance costs as well as frictions associated with data use. This also applies when the structure of data needs to be changed as part of an upgrade process. The predictability of structured data allows for more accurate resourcing of ongoing costs as well as clear planning and documentation of upgrades to data and business processes.[16] If data is published to a data standard, then many of the complexities of implementation will have already been considered, reducing initial development costs. The published data will also benefit from an existing set of documentation and technical tools.
To illustrate, in Slovakia – one of the early implementers, therefore not having the benefit of lessons learned from established practices – “the development of a new register cost around EUR 330,000”, and “[t]he yearly operating costs are estimated to be around EUR 33,000”.[17] Similarly, a study in the European Union estimated the operational costs for member states to make BO data available as structured data to be EUR 50,000 and 4-10 staff in full-time employment per year.[18] To contextualise these costs, a post-implementation valuation of user benefits in the UK concludes that, “in aggregate, the annual user benefits of CH data are estimated to be between £1 billion and £3 billion per year. This is likely to be an underestimate as it only includes benefits for Companies House Service (CHS) users.” Different modes of access made possible by publishing the information as structured data were noted as generating the greatest user value.[19]
Using beneficial ownership data
Structured BO data is quicker and cheaper to use for the public and private sector, reducing the costs of accessing critical information and of linking BO information to other datasets and systems. To illustrate, the cost for law enforcement to access unstructured information was noted as a significant motivation behind increasing BOT in Canada.[20] Where structured BO data is available, this can be readily integrated into, for example, in-house or outsourced onboarding, due diligence, or regulatory technology services. In research conducted by OO on the use of BO data by financial institutions, one bank estimated the cost of handling unstructured PDF BO reports from one jurisdiction to be “an extra EUR 7 on top per review” for customer due diligence processes, in comparison to a jurisdiction that makes its BO information available as open, structured data. This was noted to be “a big cost for the bank”, as the bank conducts thousands of reviews per year.[21]
Realising policy benefits
Structured BO data can also reduce the cost of realising policy benefits by facilitating interoperability. For example, when data is structured according to the same data standard, it provides a common language that enables different systems to speak together and can reduce the cost of realising policy aims. Additionally, these policy aims may generate economic benefits.[22] These benefits usually accrue outside the implementing agency and therefore require a holistic approach to assessing the costs and benefits of structuring data and budgeting.[23]
Structured data facilitates interoperability in multiple ways. Firstly, technical interoperability – for example, using a data standard to publish BO data – can allow tools and analysis built in one context to be reused in another, reducing costs and development times. By publishing data to BODS, Armenia was able to use the OO BODS visualisation library to automatically generate diagrams of ownership structures, thereby rapidly deploying advanced functionality in the first version of its register (see Box 5).[24] Similarly, Bluetail, a prototype tool for linking BO and procurement data, is available in several languages and has been used in Indonesia, Kenya, and South Africa (see Figure 2).[25]
Box 5. Structuring beneficial ownership data in Armenia
In its implementation of a central BO register, Armenia has moved from collecting and publishing unstructured BO data to publishing structured data in line with BODS for use across government and beyond.
Armenia’s implementation began by piloting requirements to disclose BO data for companies operating in its extractive sector. This pilot programme was used to develop and test systems for the collection and publication of data from a high-risk sector before gradually expanding the requirements to companies in all sectors of the economy by the end of 2023.
To expedite data collection and publication for the pilot in 2020, Armenia opted to collect BO information via paper forms as an interim step whilst finalising the upgrades to its registry software. These paper forms were then converted into PDF format and published online. Though this permitted some limited analysis of the disclosures by media organisations, the absence of structured data limited its utility and made carrying out any checks on the information disclosed significantly more laborious.
In September 2021, Armenia published its first structured data, becoming one of the first countries to publish data using BODS by incorporating the standard into the design of its register software.[26]
Although Armenia is still dealing with some teething issues, the adoption of the data standard will permit new uses of its BO data and enables the country to incorporate different tools developed for BODS directly into its register. For example, the BODS data visualisation library enables users to easily produce ownership graphs from the data and facilitates the understanding and analysis of company structures.[27] From late 2023, Armenia also plans to draw structured BO data from the State Register into its procurement systems as a means of tackling corruption, collusion, and bid rigging during government tender processes.
Figure 2. An unstructured beneficial ownership declaration from 2018 (left) versus a structured beneficial ownership declaration as shown through the Beneficial Ownership Data Standard data visualiser on Armenia’s beneficial ownership data portal in 2022 (right)
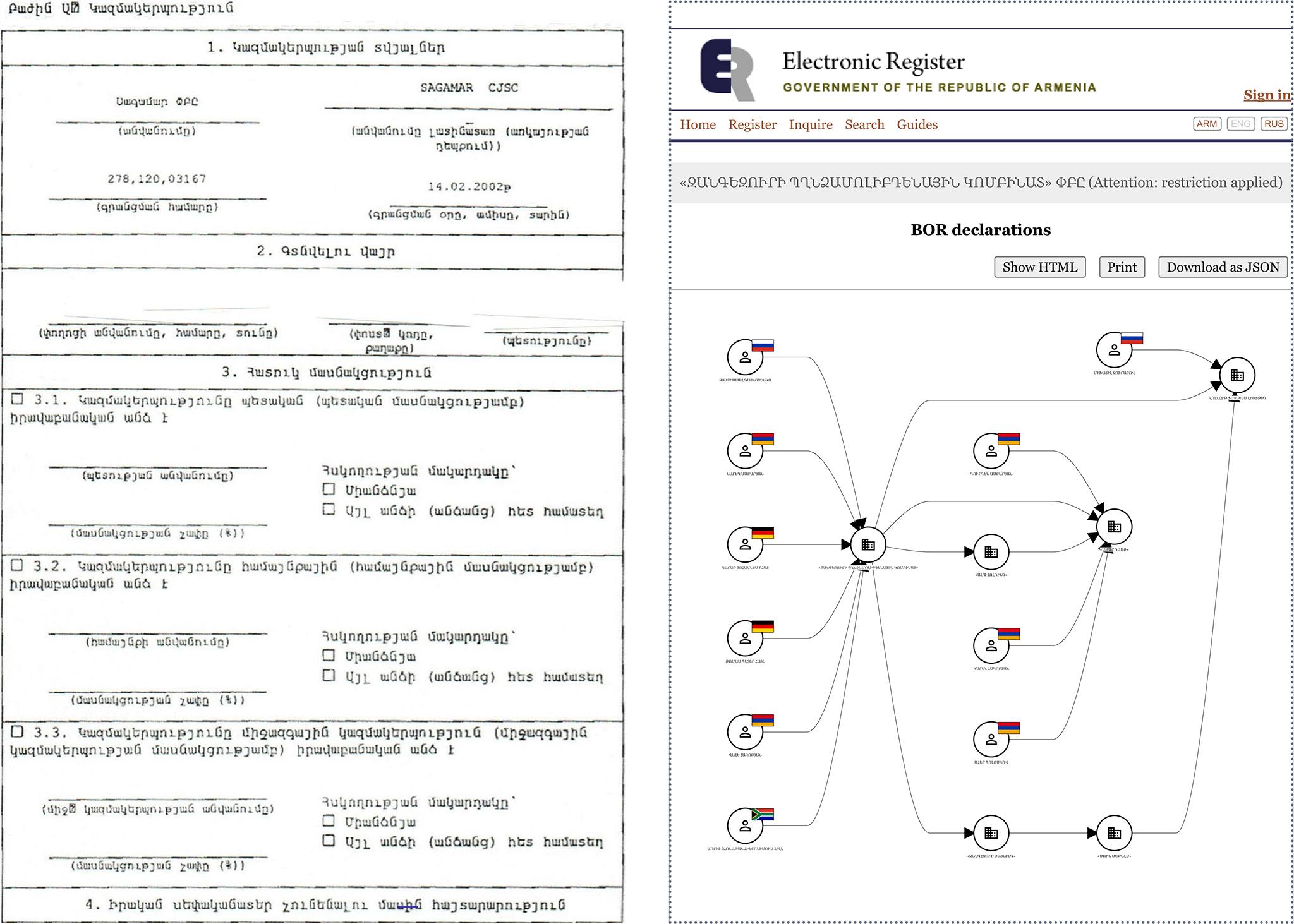
Source: www.e-register.am[28]
Figure 3. Bluetail: Combining structured beneficial ownership data and structured contracting data
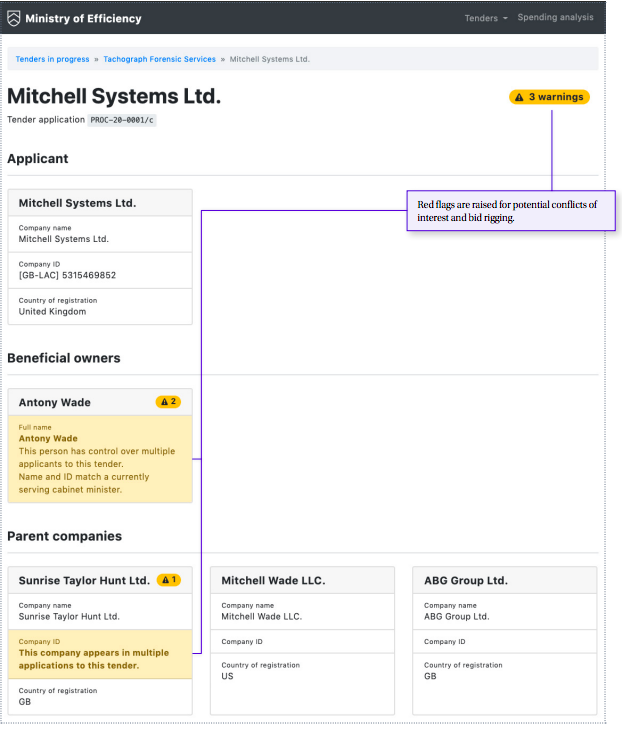
OO has built on a prototype developed by mySociety and Spend Network called Bluetail, which shows how structured data published to BODS and data published to the Open Contracting Data Standard are interoperable. These datasets can be combined to automatically raise red flags for corruption and collusion risks when procurement officers screen tenders.[29] Source: bluetail.herokuapp.com.
Structured data also improves contextual interoperability. For example, whilst a single global identifier for legal entities may improve technical interoperability, adopting a common method of assigning and disambiguating company identifiers – such as the approach developed by org-id.guide[30] – may prove more pragmatic and result in datasets that are more interoperable in practice. The Organisation for Economic Co-operation and Development’s Common Reporting Standard for tax information is another example with a high level of contextual interoperability.[31] Having high levels of contextual interoperability allows resources otherwise spent on cleaning data to be spent on analysing data. The bulk of artificial intelligence effort in corporate data is currently spent on disambiguating data. Policy makers are in a position to work on the coordination problem that would make this less necessary. More mature and established, but closely related, fields provide a useful model for building a shared infrastructure using interoperable and structured data as the baseline.
Compliance with disclosure requirements
Structured data is cheaper to produce for declaring companies’ beneficial owners and central registers. A central register can provide a standardised way of declaring information – for example, in an online form – around which companies can standardise internal processes and procedures. To make submitting compliance easier and to reduce the submission of errors, Armenia also uses the BODS visualisation library in its declaration process (see Box 5). Unstructured data, by contrast, may be collected in a variety of formats, using a variety of bespoke processes that may require specialist expertise. Registers that verify incoming information can use structured data to cross-reference with other datasets rather than solely relying on expensive manual checks, as with unstructured information.[32]
Greater policy impact
Structured BO data is more likely than unstructured information to achieve BOT policy objectives, as the advantages discussed above make it a better option for achieving policy outcomes. BO information is rarely used in isolation to achieve specific policy goals, and it is often most valuable when combined with other datasets. To be useful, data on beneficial ownership needs to be contextualised with data on the identities or activities of companies or natural persons. Structured data makes it possible to link BO information to other datasets by joining on common fields.
BO data can be linked to other BO datasets from other jurisdictions to give a more complete view of transnational ownership structures. Beneficial ownership can be exercised through complex structures that include multiple jurisdictions, particularly in cases of corruption and money laundering. In a World Bank study of around 150 grand corruption cases, nearly all relied on corporate vehicles to conceal ownership, and the majority featured transnational structures.[33] The details of such structures will not always be available from the disclosures of a single jurisdiction, and to make sense of the cross-jurisdictional nature of company ownership it is often necessary to join multiple BO datasets from different jurisdictions or to combine BO data with data on legal ownership. Interoperable data allows the information from multiple registers to be easily combined. In the World Bank study, transnational investigations and building transnational cases were flagged as two priorities to combat the misuse of corporate vehicles: “solving a transnational corporate vehicle misuse scheme is like putting together a jigsaw puzzle, with investigators in different jurisdictions each holding separate pieces of the puzzle. To complete the puzzle, an investigator needs to have access to all the pieces.”[34] Gaining visibility on transnational ownership structures will save resources that can be redirected in other areas of investigation (see, for example, Box 6).
BO data can also be combined with other datasets about individuals or legal entities for other purposes. For example, datasets relating to procurement and beneficial ownership can be linked through the use of common identifiers for legal entities.[35] Additionally, BO data can be combined with political campaign financing data to safeguard electoral processes.[36] Whilst datasets can still be linked without shared identifiers, it will be necessary to reconcile the data to distinguish which records refer to the same legal or natural person, a time-consuming and uncertain process.[37] Identifiers for real-world entities are therefore an essential requirement for the effective implementation of structured data (see, for example, Box 6).
Box 6. OpenSanctions and OpenScreening: Combining beneficial ownership and sanctions data using real-world identifiers
Sanctions against individuals or companies on the United States (US) Office of Foreign Assets Control list apply not just to the entity itself, but also to all companies majority-owned or controlled by that entity.[38] This provision, also adopted by some other authorities, makes visibility of full company structures and beneficial ownership an essential part of sanctions compliance.
OpenSanctions is an international database combining information on individuals and legal entities from multiple sources – including national BO registers – and linking this to sanctions and politically exposed persons lists. The project combines this information into a single dataset to help cross-check databases, detecting conflicts of interests and signs of illicit activity as well as customer due diligence in international dealings, and tracking political conflicts and sanctions policies across the world.[39] This requires deduplication and ensuring there is only one consolidated entry for each legal entity and natural person based on issued identifiers and descriptive data fields.[40] Depending on the information available, this may require manual work.[41]
OpenSanctions matches its dataset to the Legal Entity Identifier (LEI) reference data released by the Global Legal Entity Identifier Foundation (GLEIF). GLEIF has developed a global database[42] of corporations that have been issued a LEI, and also publishes information about ownership relationships between companies.[43] This allows OpenSanctions to enrich its data and identify additional relationships.[44]
For the OpenScreening project, OpenSanctions has mapped BO data from the Offshore Leaks database published by the International Consortium of Investigative Journalists (ICIJ) to the FollowTheMoney data model used by OpenSanctions and the Organised Crime and Corruption Reporting Project.[45] This data is then combined with OpenSanctions’ own database, loaded into a Neo4J graph database, and uploaded to a data visualisation platform provided by Linkurious so that people can explore and visualise the connections between sanctioned or politically exposed people and leaked BO data using open data.[46]
Figure 4. OpenScreening
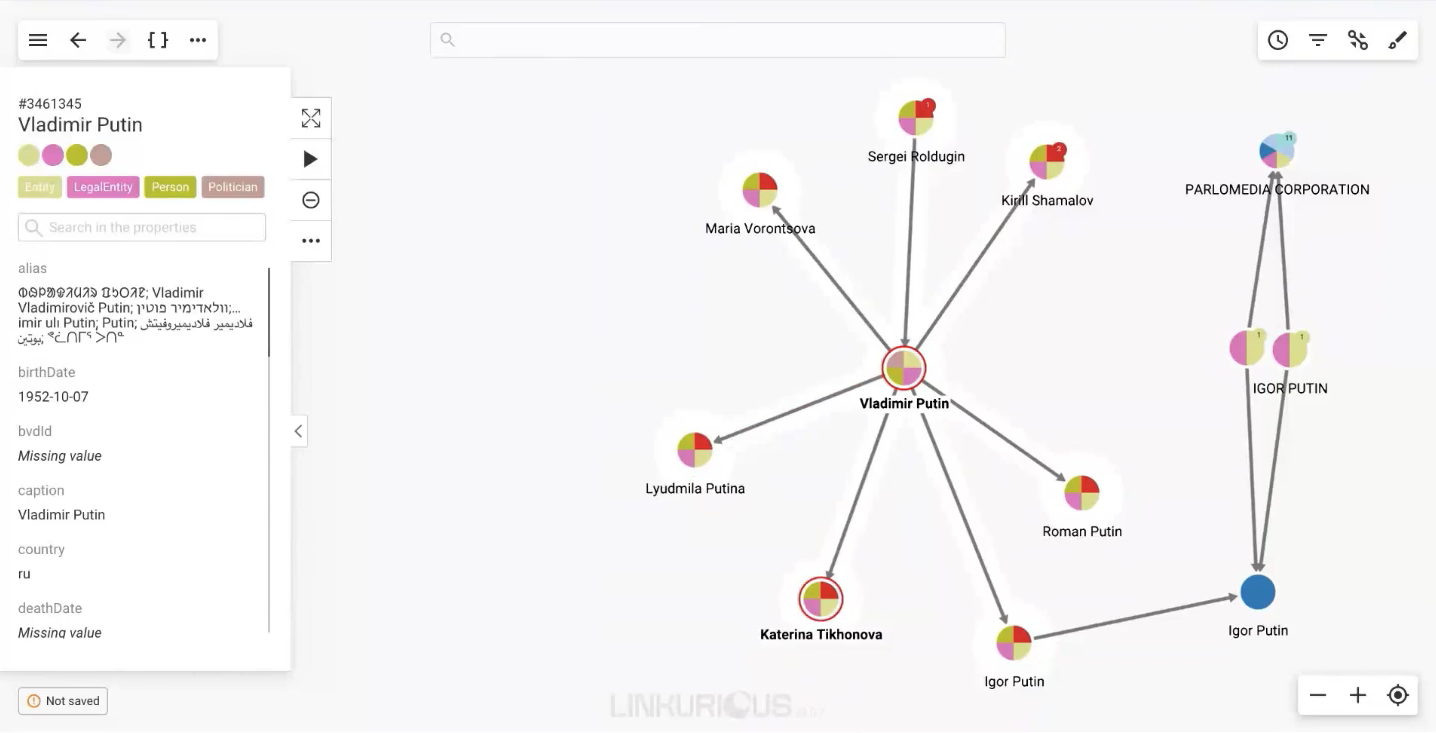
A visual example of OpenSanctions’ graph data being combined with Linkurious’ investigation software, which also plans to ingest data from the OO Register.[47] Source: resources.linkurious.com/openscreening.
The specific needs of the BOT policy area also mean that structured data has a significant advantage over unstructured data. Unstructured BO information quickly creates resource constraints that act as a barrier to action and ambition, whereas structuring BO data opens up new types of analysis and possibilities (see Figure 4). Unstructured information tends to create analysis that is resource-intensive and small in scale, which is viable in only a few contexts, such as investigations relating to grand corruption cases. Structured data allows for macro-scale and experimental analysis of entire BO datasets at low cost. This opens up different kinds of policy impact that are impossible without structured data, for example: examining national security risks relating to BOT across specific sectors of the economy; statistical analysis of the effectiveness of policy interventions; or the development of red-flagging procedures to better target manual investigations. In the UK, the Competitions and Markets Authority has started using BO data to analyse the concentration of ownership in specific sectors once common ownership and control are taken into account.[48] In another example, researchers used a commercial company ownership dataset (which includes beneficial ownership) to map stranded fossil-fuel assets to understand market risk in the energy transition.[49]
Structured data also enables timely access to auditable information. This is particularly relevant for law enforcement and national security purposes, as it removes the need for manual searches and requests, which risk tipping off suspects, or delaying investigations. Automated controls and access logs can ensure that confidential or restricted information is used appropriately.
Finally, structured data creates the potential for more trusted data and more resilient systems through verification and register-wide analysis. This can include automated verification of data submitted to the register; checks and challenges based on threshold-driven tests; and the collection and analysis of metadata on submissions (e.g. the use of particular company formation agents) that may reveal vulnerabilities in particular areas of the system. This also means that personal information can be shared in a way that conforms with privacy and data protection legislation by building in varying permissions for different data fields for specific data users. For example, a registrar checking a passport number with a different government agency for identity verification purposes would simply be able to receive information on whether or not the values match existing records, without sharing additional personal information.[50]
Foot note
[e] The OO register ingests data from four national BO registers. See: “Open Ownership Register – Data Sources”, Open Ownership, n.d., https://register.openownership.org/data_sources.
End notes
[6] “The companies we keep: What the UK’s open data register actually tells us about company ownership”, Global Witness, n.d., https://www.globalwitness.org/en/campaigns/corruption-and-money-laundering/anonymous-company-owners/companies-we-keep.
[7] “Learning the lessons from the UK’s public beneficial ownership register”, Global Witness and Open Ownership, November 2017, 8, https://www.openownership.org/en/publications/learning-the-lessons-from-the-uks-public-beneficial-ownership-register.
[8] “Learning the lessons from the UK’s public beneficial ownership register”, Global Witness and Open Ownership, 8.
[9] Ben Cowdock, “Tackling abuse of the Scottish Limited Partnerships needs a UK-wide money laundering reform”, Transparency International UK, 3 May 2018, https://www.transparency.org.uk/tackling-abuse-scottish-limited-partnerships-needs-uk-wide-money-laundering-reform.
[10] See, for example: David Leask and Richard Smith, “Scots shell companies used to launder £4 billion out of Russia”, The Herald, 27 March 2017, https://www.heraldscotland.com/news/15183346.scots-shell-companies-used-to-launder-4-billion-out-of-russia/; Jaccy Gascoyne, “The Use of Scottish Limited Partnerships in Money laundering Schemes”, MLROs.com, n.d., https://mlros.com/the-use-of-scottish-limited-partnerships-in-money-laundering-schemes/.
[11] Chinwe Ekene Ezeigbo, Tymon Kiepe, and Louise Russell-Prywata, “Early impacts of public registers of beneficial ownership: United Kingdom”, Open Ownership, 1 April 2021, https://www.openownership.org/en/publications/early-impacts-of-public-registers-of-beneficial-ownership-uk/.
[12] For the full case study, see: Alanna Markle, “Early impacts of public beneficial ownership registers: Ukraine”, Open Ownership, 1 February 2022, https://www.openownership.org/en/publications/early-impacts-of-public-beneficial-ownership-registers-ukraine.
[13] YouControl, “About us”, n.d., https://youcontrol.com.ua/en/about_us/#about-system.
[14] See: YouControl, “Case Studies”, n.d., https://youcontrol.com.ua/en/cases/.
[15] “ISO 8601 – Date and time format”, International Organization for Standardization, n.d., https://www.iso.org/iso-8601-date-and-time-format.html.
[16] For more information on systems and business processes in BOT, see: “Guide to implementing beneficial ownership transparency”, Open Ownership, July 2021, 13, https://www.openownership.org/en/publications/guide-to-implementing-beneficial-ownership-transparency/.
[17] Juraj Labant and Gabriel Šípoš, “The Register of Beneficial Ownership in Slovakia: Revolutionary transparency, questionable implementation, unsure benefits”, Transparency International Slovakia, June 2017, http://transparency.sk/wp-content/uploads/2017/06/Register-of-beneficial-ownership_study2017.pdf.
[18] “Impact Assessment study on the list of High Value Datasets to be made available by the Member States under the Open Data Directive”, Deloitte, EC Directorate-General for Communications Networks, Content and Technology, 30 December 2020, 128, https://doi.org/10.2759/493091.
[19] “Valuing the user benefits of Companies House data – Report 2: Direct Users”, BEIS, Research Paper Number 2019/015, September 2019, 24, https://assets.publishing.service.gov.uk/government/uploads/system/uploads/attachment_data/file/833767/valuing-benefits-companies-house-data-report-2-direct-users.pdf. For more information on measuring the economic benefits of BOT reforms, see: “Measuring the economic impact of beneficial ownership transparency”, Lateral Economics, Open Ownership, and Oxford Insights, May 2022, https://www.openownership.org/en/publications/measuring-the-economic-impact-of-beneficial-ownership-transparency-summary-report/.
[20] “B.C. Consultation on a Public Beneficial Ownership Registry”, Province of British Columbia, Ministry of Finance, January 2020, 12, https://engage.gov.bc.ca/app/uploads/sites/121/2020/01/386142-BCABO-Consultation-Document-For-Release.pdf.
[21] Paul May, “The use of beneficial ownership data by a financial service institution”, Open Ownership, Unpublished.
[22] For more information on quantifying the economic benefits of BOT, see: “Measuring the economic impact of beneficial ownership transparency”, Lateral Economics et al.
[23] Tymon Kiepe, “Making central beneficial ownership registers public”, Open Ownership, 4, https://www.openownership.org/en/publications/making-central-beneficial-ownership-registers-public.
[24] “openownership/visualisation-tool: A visualisation library for beneficial ownership structures”, GitHub, n.d., https://github.com/openownership/visualisation-tool.
[25] Steve Day, “Transforming procurement systems, one prototype at a time”, Open Ownership, 18 December 2020, https://www.openownership.org/blogs/tps-prototyping.
[26] “Armenia and Latvia become first countries to publish data in line with the Beneficial Ownership Data Standard”, Open Ownership, 15 September 2021, https://www.openownership.org/en/blog/armenia-and-latvia-become-first-countries-to-publish-data-in-line-with-the-beneficial-ownership-data-standard.
[27] “openownership/visualisation-tool”, GitHub.
[28] “Sagamar CJSC – Electronic Register”, Government of Armenia, 2018, https://www.e-register.am/am/companies/1267708/beneficiary.pdf; “BOR declarations: «ԶԱՆԳԵԶՈՒՐԻ ՊՂՆՁԱՄՈԼԻԲԴԵՆԱՅԻՆ ԿՈՄԲԻՆԱՏ» ՓԲԸ – Electronic Register”, Government of Armenia, 25 June 2021, https://www.e-register.am/en/companies/1340197/declaration/c51e08a7-6fdb-4ab7-a55c-c74a68a8f54c?diagram=1.
[29] For more information, see: Day, “Transforming procurement systems, one prototype at a time”. For more information about using BO data in procurement, see: Tymon Kiepe and Eva Okunbor, “Beneficial ownership data in procurement”, Open Ownership, 11 March 2021, www.openownership.org/en/publications/beneficial-ownership-data-in-procurement.
[30] “org-id.guide – list locator (Alpha)”, org-id.guide, n.d., https://org-id.guide/about.
[31] See: “Common Reporting Standard (CRS)”, OECD, n.d., https://www.oecd.org/tax/automatic-exchange/common-reporting-standard/.
[32] For more information on how structured data can help with various approaches to verification, see: Tymon Kiepe, “Verification of beneficial ownership data”, Open Ownership, 7 May 2020, https://www.openownership.org/en/publications/verification-of-beneficial-ownership-data.
[33] Emile van der Does de Willebois, Emily M. Halter, Robert A. Harrison, Ji Won Park, and J.C. Sharman, The Puppet Masters: How the Corrupt Use Legal Structures to Hide Stolen Assets and What to Do About It (Washington DC: The World Bank, 2011), 52, 121, https://star.worldbank.org/sites/star/files/puppetmastersv1.pdf.
[34] Van der Does de Willebois et al., The Puppet Masters: How the Corrupt Use Legal Structures to Hide Stolen Assets and What to Do About It, 9.
[35] “Beneficial ownership information”, Open Contracting Data Standard, n.d., https://standard.open-contracting.org/latest/en/guidance/map/beneficial_ownership.
[36] Tymon Kiepe, “Using beneficial ownership data for national security”, Open Ownership, 17 December 2021, 18-19, https://www.openownership.org/en/publications/using-beneficial-ownership-data-for-national-security.
[37] Steve Day, “Reconciling beneficial ownership data”, Open Ownership, 17 August 2020, https://www.openownership.org/blogs/reconciling-beneficial-ownership-data; Jason Arterburn, Erin D. Dumbacher, and Page O. Stoutland, “Signals in the Noise: Preventing Nuclear Proliferation with Machine Learning & Publicly Available Information”, Nuclear Threat Initiative and Center for Advanced Defense Studies, 12 January 2021, https://www.nti.org/analysis/reports/signals-in-the-noise.
[38] “Revised Guidance on Entities Owned by Persons Whose Property and Interests in Property Are Blocked”, US Department of the Treasury, 13 August 2014, https://home.treasury.gov/system/files/126/licensing_guidance.pdf.
[39] “About this project”, OpenSanctions, n.d., https://www.opensanctions.org/docs/about.
[40] “Identifiers and de-duplication”, OpenSanctions, n.d., https://www.opensanctions.org/docs/identifiers.
[41] “How we deduplicate companies and people across data sources”, OpenSanctions, 11 November 2021, https://www.opensanctions.org/articles/2021-11-11-deduplication/.
[42] “Global LEI Index”, Global Legal Entity Identifier Foundation, n.d., https://www.gleif.org/en/lei-data/global-lei-index.
[43] “Level 2 Data: Who Owns Whom”, Global Legal Entity Identifier Foundation, n.d., https://www.gleif.org/en/lei-data/access-and-use-lei-data/level-2-data-who-owns-whom.
[44] “ Legal Entity Identifier (LEI) Reference Data”, OpenSanctions, n.d., https://www.opensanctions.org/datasets/gleif/.
[45] “opensanctions/icij-offshoreleaks: Converter for ICIJ Offshore Leaks data into FollowTheMoney format used by OpenSanctions”, GitHub, n.d., https://github.com/opensanctions/icij-offshoreleaks.
[46] “OpenScreening”, Linkurious, n.d., https://resources.linkurious.com/openscreening; “opensanctions/offshore-graph: Loading OpenSanctions into Neo4J and Linkurious”, GitHub, n.d., https://github.com/opensanctions/offshore-graph.
[47] At the time of writing, OO is working with the OpenSanctions team to include the BO data from the OO Register in this project.
[48] Open Ownership, “Open Ownership technology showcase #4”, YouTube video, 1:03:14, posted 18 May 2022, https://www.youtube.com/watch?v=ipKkRX8vpZg.
[49] Gregor Semieniuk et al., “Stranded fossil-fuel assets translate to major losses for investors in advanced economies”, Nature Climate Change 12, (26 May 2022): 532-538, https://doi.org/10.1038/s41558-022-01356-y.
[50] For more detail on creating trusted BO data through verification, see: Kiepe, “Verification of beneficial ownership data”.
Next page: Operationalising structured beneficial ownership data