Spotting red flags in beneficial ownership datasets
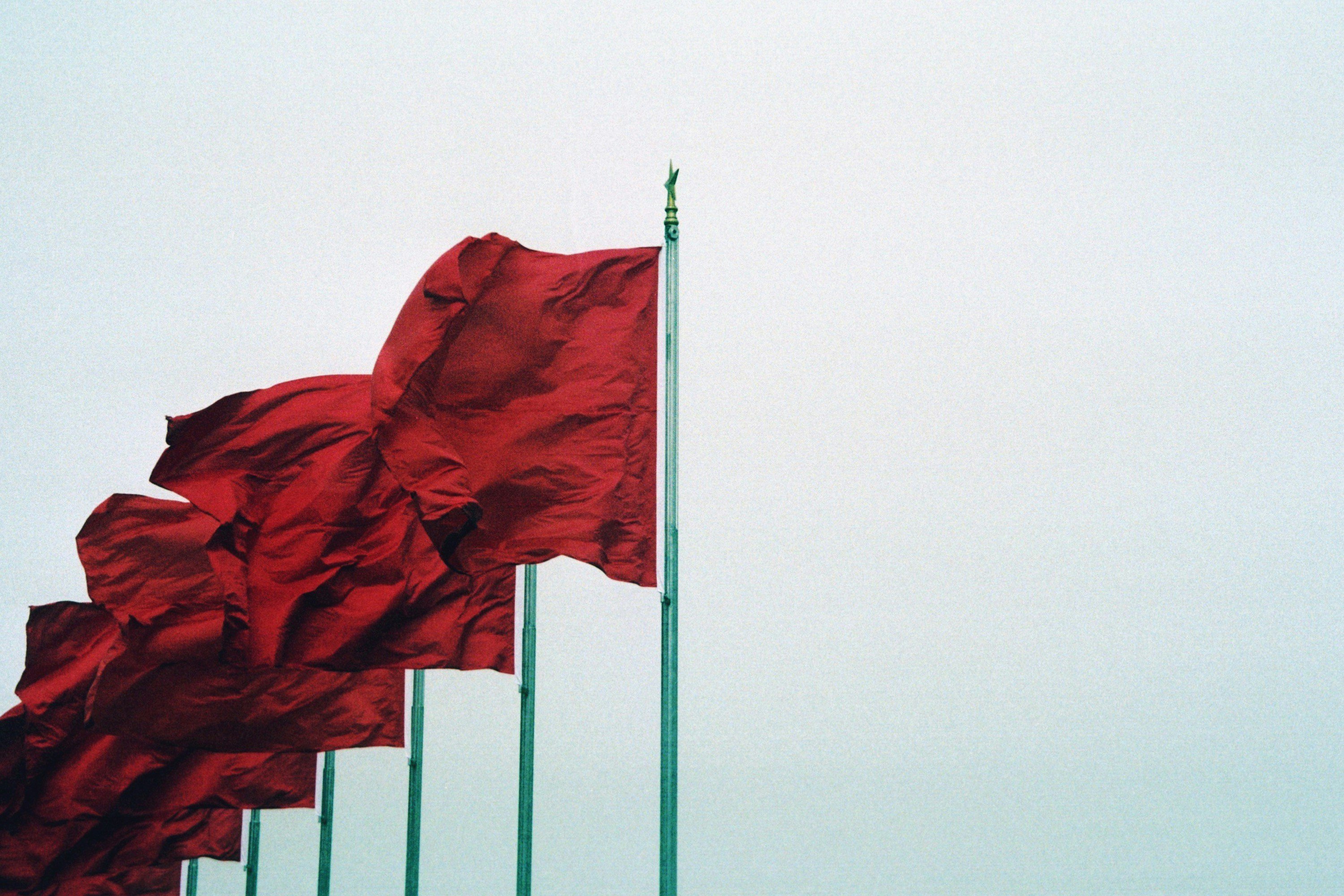
Photo by Zachary Keimig on Unsplash
This blog post talks about one of the key benefits of improving the quality of beneficial ownership (BO) data: bulk analysis to find patterns or “red flags”.
Red flags relating to the ownership or control of corporate vehicles can be spotted more easily when BO data is structured, for example, in line with the Beneficial Ownership Data Standard (BODS). The detail on this was set out in the 2022 Open Ownership briefing on structured and interoperable data.
Open Ownership and our technical partners at Open Data Services sought to put this into practice by showcasing how data users can write queries to spot a number of red flags in BODS datasets.
The questions we sought to answer were:
- How many entities declare that they have no beneficial owners or that their beneficial owners are unknown?
- How many entities have ownership networks which involve natural persons or entities in countries on the EU list of non-cooperative tax jurisdictions?
- How many entities have complex ownership chains? (defined here as 5+ observable layers of ownership)
- How many entities were founded and subsequently dissolved within a year?
We chose these questions after examining a wide range of research conducted by civil society groups, academics and investigative journalists in recent years.
To attempt to answer these questions, we examined open BODS data from the United Kingdom, which Open Ownership republishes on a regular basis via our BODS data analysis tools. We also harnessed Deepnote’s data science platform and the NetworkX Python package for analysing complex networks.
The results of our investigation can be found in this public red flags analysis Deepnote notebook and – where possible – we have added new queries to our BODS analysis Github repository which already provides dozens of example queries that analysts can use to examine BODS data. Those queries are also used by our analysis notebooks and dashboards for BODS data.
This work taught us more about the importance of understanding:
- How data can be extracted
- How the countries where entities and people are based are represented in the data
- How to link entities and people to the rest of their ownership chains
Our investigation showed how it is not always as easy as one would like to answer the above four questions. This in itself is invaluable as we improve our technical ability to map data sources to BODS. And, in the coming months, we plan to share more about what we learned during this process and how we use those insights to shape the development of BODS.
Related articles and publications
Publication type
Blog post
Topics
Beneficial Ownership Data Standard
Sections
Implementation
Open Ownership Principles
Structured data